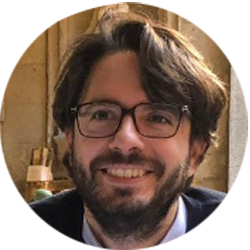
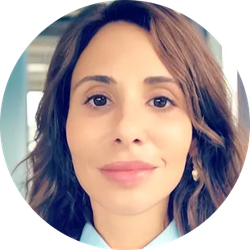
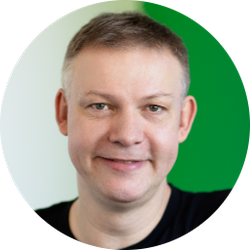
Knowledge Graphs and Semantic Layers to support Enterprise Information Architecture for AI
A Talk by Andrea Gioia , Sofus Macskassy and Jessica Talisman
About this Talk
Most enterprise organizations are exploring or using knowledge graphs. Meanwhile, knowledge graphs are at the top of the Garter AI Hype Cycle, although Knowledge Graphs are much more than hype, these practices involve mature technology used in large scale deployments. Knowledge Graphs are being deployed in a large variety of industries, including: finance; healthcare, pharma, and life sciences; manufacturing; energy; and throughout the public sector.
That said, most enterprise data management practices rely on more traditional relational technology. Many such organizations tend to have invested in practices such as MDM and data catalog – often where initiatives had failed before, being too slow, too long of an effort, or difficult to maintain. More recently, enterprise architecture teams are hearing about data mesh and data products. How do any of these practices align with knowledge graph work?
Emerging notions of semantic layer address these issues. While we’ve heard for years that an appropriate strategy is to place data into a data warehouse / data lake / data lakehouse – the reality of enterprise information architecture is that each domain within an organization will have its own specific definitions and semantics.
Of course, merely dumping datasets into a relational data lake generally obscures the domain-specific semantics and downstream use cases. Instead we can leverage knowledge graphs to describe the interfaces needed between different domains, plus related technologies such as entity resolution to perform merges among multiple datasets more effectively and generate graph elements. Moreover, much depends on an organization’s culture, in terms of which approaches work best for connecting data and abstracting this into layers.
Overall, these more recent practices help improve data quality and provenance, they can help improve security practices, and definitely enhance the AI apps downstream. While information retrieval practices (search, recsys, question/answer) have been dominant for many years, now methods of reasoning become more of a priority.
How do we find people who are fluent in these areas of work? Fortunately, library science comes to the rescue, with a rich portfolio of solutions to apply for harmonizing across vastly differing metadata and building a semantic layer – particularly for how to engage a wide variety of groups ranging from data governance to AI engineers.
Key Topics
- What are Knowledge Graphs good for?
- Supporting technologies
- How can I get started?
- Which roadblocks should I watch out for?
Target Audience
- Chief Data Officers
- Enterprise Architects
- Data Governance teams
- Data Science teams
- Data Modelers
- Technical Managers
Goals
- Explore the interplay between machine learning and knowledge based technologies
- Answer questions that matter
- How can those approaches complement one another, and what would that unlock?
- What is the current state of the art, how and where is it used in the wild?
- What are the next milestones / roadblocks?
- Where are the opportunities for investment?
Session outline:
- Introduction
- Meet and Greet
- Setting the stage
- Why Knowledge Graphs?
- What kind of problems can we solve by using knowledge graphs?
- What kind of problems are not best solved with knowledge graphs?
- How are knowledge graphs any better than relational technology?
- How do knowledge graphs address the problem of silos?
- What are some real world examples of knowledge graphs in the enterprise?
- Deploying a Knowledge Graph in Your Enterprise
- Where does the data come from to populate knowledge graphs?
- Who are the major vendors for knowledge graph technology?
- What are some common technology stacks used in knowledge graph deployments?
- How do the different implementations compare?
- What skills are required to deploy a knowledge graph application?
- What is the breadth and depth of tooling for knowledge graphs?
- How good are knowledge graph visualization tools?
- What is a Semantic Layer?
- Do we proceed top-down, bottom-up, or some other way?
- Projects had failed before (too slow, too long of effort, hard to maintain)
- Verifiability and discovery of new concepts.
Format
- Extended panel
- Expert discussion, coordinated by moderator
- 2 hours running time
- Running time includes modules of expert discussion, interspersed with modules of audience Q&A / interaction
Level
- Intermediate
Prerequisite Knowledge
- A basic understanding of Knowledge Graphs will be helpful.
- Some familiarity with using enterprise data systems, such as a data warehouse or data lake.